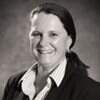
Using Advanced Analytics to Predict the Onset of a Cytokine Storm
A team of Teradata data scientists, clinicians & engineers set out to build a model that could track and predict the onset of a Cytokine Storm. #COVID-19
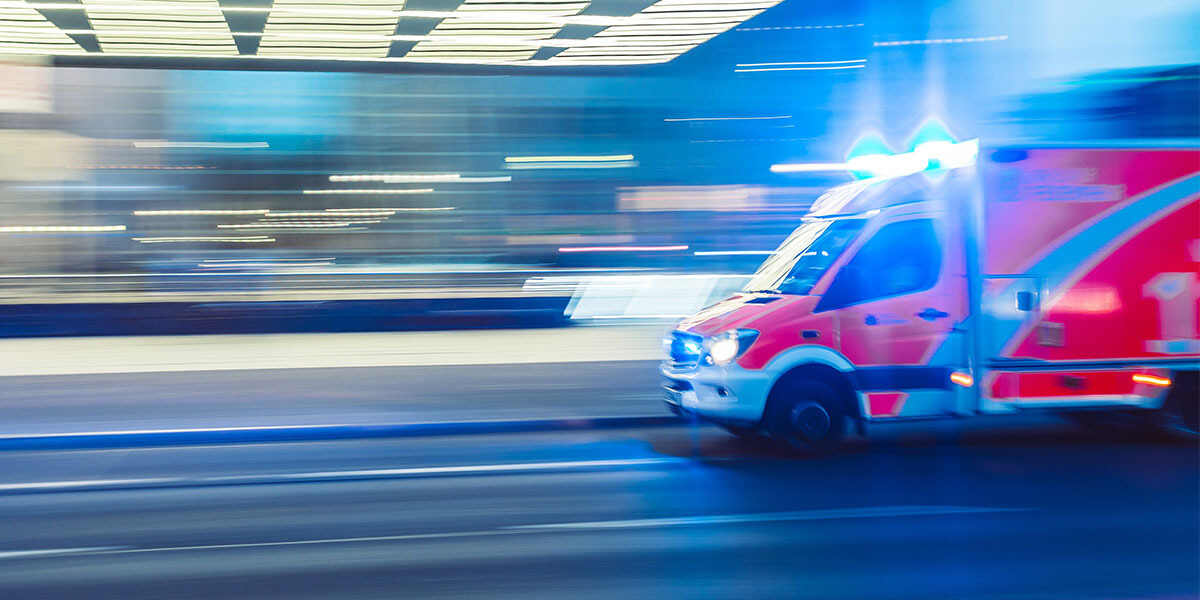
Cytokines are small proteins in the body that regulate the immune system. A cytokine storm causes these proteins to fail in properly regulating the immune system. The immune system goes into over-drive and begins attacking the body. There are many pathways into the cytokine storm from a variety of conditions: Sepsis, organ transplant rejection, viruses like COVID-19 and many others. As the storm builds, there are variables that can be analyzed to understand its development, size and pathway. It is very similar to the process to predict a hurricane or tornado. Prior to a hurricane/tornado there are notable changes in variables: Barometric pressure, temperature, wind, rain, etc., that can be assessed to understand the size and pathway of the impending storm.
Teradata recently hosted an internal COVID-19 Hackathon that addressed solutions to help the fight against the virus. A team of data scientists, clinicians and engineers set out to build a model that could use a similar conceptual process to the national weather service’s storm tracking of hurricanes.
The Teradata team used a public data set that included robust variables included in medical notes, devices and electronic medical records. The dataset used is MIMIC III, or Medical Information Mart for Intensive Care III (MIMIC-III) ICU datasets, which is a rich dataset with the key variables, clinical metrics and granular time-recorded events, medications, procedures, etc., of patients admitted in hospital during ICU stay.
The tools and techniques used were text analytic data mining, machine learning, statistical modeling, linear-regression and predictive analytics. Teradata’s Vantage platform and tools were used for the model development.
The team reviewed 80+ variables spanning vital signs, body systems and labs including, but not limited to: temperature, blood pressure, heart rate, SAO2, CRP, Ferritin, D-Dimer, etc. The variables were found using text analytics and a variety of other techniques.
The goal of the model was to determine when the cytokine storm was in full force and to understand events that precipitate the storm. By leveraging Teradata Vantage’s time-series, pathing and machine learning tools the team was able to illustrate, visualize and predict paths/patterns and events leading up to the storm so that the scientists and clinicians could start to predict the timing of the impending storm.
The variables were organized by patient on a continuous time-series framework and by body system (lungs, circulation, kidneys, etc.) to predict the storm as far as possible in advance. The model assigned a cytokine risk score for every patient in the data and it was recalculated whenever variables were updated. The model noticed that as a storm approaches the individual cytokine score became stormy and fluctuated as body systems began to be impacted and or fail. This is similar to an approaching hurricane where there are changes in temperature, barometric pressure and clouds.
In COVID-19 Cytokine Storms there has been a focus on specific variables lab tests: Creatine (kidneys), C-Reactive Protein or CRP (inflammation marker) ferritin (iron), D-Dimer (fibrin or clotting). These variables are typically abnormal when the storm is imminent. As our team reviewed all the events/variables, we went upstream to understand those that were first to be impacted and those that were impacted right as the storm was hitting.
Our team determined -- through machine learning, time-series and pathing -- that focusing on a broader set of variables allowed for our model to predict the onset of a Cytokine Storm with 67% accuracy up to 30 hours in advance. The model was able to identify those who were in the storm and the events that led up to it. In our model we identified various demographics or ethnicities that were most severely impacted by the cytokine storm and corresponding mortality rates.
The team felt that the broader data set of variables provided the ability to predict further in advance rather than focusing only on the smaller set of variables used most recently in COVID-19 to predict the actual storm.
In recent news and publications there has been a focus on specific variables like CRP, ferritin, creatinine and D-Dimer. Our model and experts suggest by limiting the variables it reduces the visibility of the approaching storm.
Teradata’s Cytokine Storm Tracker Model can predict the size, speed and pathway of a patient who’s fighting this storm regardless of whether it is caused by COVID-19, sepsis, influenza or auto-immune disorders. The model identifies the onset of a storm 30 hours in advance of it hitting which can provide earlier interventions, preparedness of staffing and supplies (ventilators, ICU beds, etc.), ultimately improving outcomes and saving lives of those who are facing this battle.
Teradata is working with several of our healthcare clients interested in testing and deploying this model to help fight the Cytokine Storm battle.
Restez au courant
Abonnez-vous au blog de Teradata pour recevoir des informations hebdomadaires