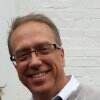
3 Factors to Consider When Evaluating Self-Service Analytics
What is the value of self-service analytics in your organization? What personas provide the most value & where should a business focus its resources? Read more.

The Value and Challenges
In Part 1 of our Is Self-Service Analytics Sustainable? series, we discussed the various types of personas, their skillsets and needs for self-service analytic capabilities. In Part 2, we will discuss the value of self-service analytics and the importance of balance between exploration and operational efforts.A key consideration in understanding the value of self-service analytics hinges on the personas discussed in part 1, i.e. General Consumers, Data Analysts, Citizen Data Scientists and Data Scientists. Three of the personas have skillsets which enable them to work outside the boundaries of traditional BI tools. They all have a desire to explore data in ways which provide new insights to questions never asked before. Their skillsets differ, which limits their data reach. But does one persona provide more business benefit to an organization? And based on that, where should an organization focus their resources? Here are three factors to consider:
Now that the self-service analytics “genie” is out of the bottle, it can’t be put back. Data sprawl is at a tipping point within most organizations and self-service analytics is stoking the fire.
-
Data Usage:
Percentages of personas differ in each organization. In general, 80-90+% of the business users fall into the General Consumer category, 10-15+% fall into the Data Analyst category, <5% fall into the Citizen Data Scientist category and typically <1% fall into the Data Scientist category. Again, these numbers vary by organization, but the point of the percentages provides insight into how each persona is leveraging high-quality, trusted data. General Consumers and Data Analysts together make up 90-95% of the user base and require trusted data for their analytic needs. Citizen Data Scientists and Data Scientists typically make up less than 5% of the user base and leverage trusted data where possible to reduce their data wrangling efforts, which can also improve the quality of their overall results.
-
Business Acumen:
Business knowledge also varies by persona. General Consumers, Data Analysts and Citizen Data Scientists typically have deep business knowledge in the business areas they represent. The Data Scientist may also have deep business understanding within a specific business area, but many times work closely with business users to understand the business problem they are requested to solve.
-
Skillset Availability:
Organizations today have extensive experience in traditional data analytics, i.e. descriptive and diagnostic analytics. Technology and skillset advancement in these areas are ubiquitous. General Consumers and Data Analysts have an excellent handle in this space, and organizations can readily acquire these valuable skillsets.
Predictive and prescriptive analytics have also been around for some time. Technology advancements have greatly improved over recent years, no longer requiring the use of sample datasets and enabling the advanced analytics of non-traditional data, e.g. voice and video. However, large data volumes, high velocity of data and data variability add to the complexity of leveraging the tsunami of new data. Data Scientists have the deep and diverse technical chops to deal with these challenges, but they are few and the demand is high. Citizen Data Scientists are being developed within organizations to begin filling this gap. They are learning to create and test machine learning models. They may not have the mathematic expertise to determine if a model is overfitting or underperforming, so they may work with a Data Scientist to validate and tweak their models before being implemented into a production environment.
These factors help provide a window into the challenges of balancing the needs of user personas within an organization. The bulk of users provide critical day in and day out support. But what about self-service analytics done by Data Analysts, Citizen Data Scientists and Data Scientists? Their value is harder to quantify, because they don’t know what they don’t know. When they do find valuable nuggets of information they can have significant impact to an organization. Sometimes these are one-and-done efforts, which impact policies, procedures or organizational structure. Once results are understood, action can be taken. Other analytic efforts need to be rerun on a regular basis and eventually are operationalized, becoming an integral part of the overall analytic ecosystem. Their work is critical to finding new markets, improving operational efficiencies and growing existing market share.
Most organizations strive to manage both, but often struggle with how to balance self-service analytics (which focus on speed-to-insight) with operational analytics (which considers total-cost-of-ownership). According to Harvard Business Review, a staggering $3.1 trillion of cost per year, in the U.S. alone, is due to the “Hidden Data Factory,” citing the following statistics of time spent by various users:
- As much as 50% is spent on hunting for data, finding and correcting errors, and validating data sources
- Often 60% or more is spent on cleaning and organizing data
Some argue that self-service analytics has taken us backwards. In the eWeek article, Why Self-Service Analytics Has Gone Backward-and What To Do About It, it identifies increasing data redundancy, under-performance of desktop tools on server-size datasets, incomplete data lineage and governance, IT only accessible data lakes, NoSQL file structures, the rise of microservices requiring IT involvement and the overall multi-platform complexity as key contributors.
Now that the self-service analytics “genie” is out of the bottle, it can’t be put back. Data sprawl is at a tipping point within most organizations and self-service analytics is stoking the fire. Organizations, both business and IT, desire to simplify the analytic platform, minimize data movement, improve data quality and better enable the analytic user community. It’s not an “either-or” proposition, structured vs semi-structured data, data warehouse vs data lake, BI vs AI, exploration vs operations, business vs IT. These all must work seamless together. But how?
Restez au courant
Abonnez-vous au blog de Teradata pour recevoir des informations hebdomadaires